Cities are responsible for a significant proportion of global energy consumption and carbon dioxide emissions, making them a major contributor to climate change. As Europe seeks to lead the transition to clean energy and reduce its carbon footprint, cities and the building sector have a crucial role to play in achieving this goal. Positive Energy Districts (PEDs), defined as urban neighbourhoods with net-zero annual energy imports and net-zero carbon emissions, are an essential part of a comprehensive approach to sustainable urbanisation. Cities need to shift their focus from individual building solutions to PEDs to ensure efficient operation.
Integrating local renewable energy sources (RES) and excess/waste heat (E/WH) is critical to achieving this net-zero goal. However, due to the intermittent nature of these energy sources and the mismatch between renewable energy production and demand, integration can be challenging. Integration can be facilitated through demand response (DR) strategies, which consist of modifying the electricity consumption curve to better match supply and demand. Several tools can be used at the district level, including the use of energy storage (electric vehicles and batteries, and thermal energy storage), the use of thermal inertia of buildings, and the use of smart grid technologies and advanced control algorithms.
The InterPED project aims to enable optimal energy management of PEDs through an integrative Cross-Vector Optimisation (CVO) approach across energy vectors and sectors. This CVO platform acts as a high-level controller within districts, using optimisation algorithms and predictive modelling to optimise energy use. It coordinates the interaction between different energy systems, including heating, cooling, electricity, and transport, to ensure efficient use of resources and maximise overall system performance. This approach facilitates the efficient use of local RES and ensures optimal interaction with the grid, promoting grid stability and resilience.
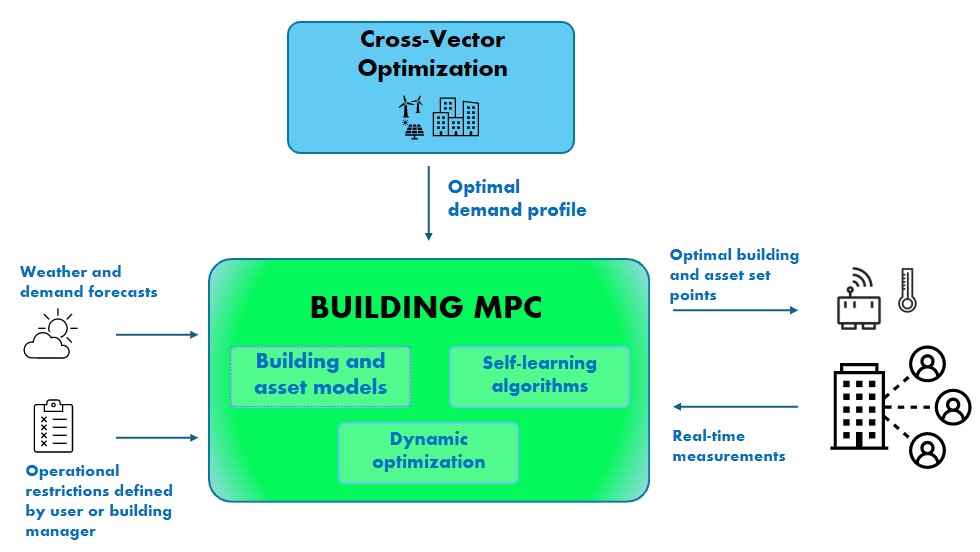
In contrast, Model Predictive Control (MPC) serves as the field-level controller in InterPED, focusing on optimising energy management at the individual building or asset level. MPC uses predictive models of the system, typically using real-time measurements, weather/demand forecasts and historical data to predict system behaviour. These models allow MPC to predict future conditions and optimise control actions accordingly. In essence, MPC works by solving a dynamic optimisation problem at each time step, considering the current system state, forecasts, and predefined objectives, to determine the optimal control actions over a finite future horizon. Once these optimal actions are calculated the MPC applies them to the system and the process repeats iteratively.
Working with the CVO platform at a higher level, MPC translates the optimal demand profile into specific control set points (heat and cold production equipment, HVAC equipment, indoor comfort conditions, etc.) that are aligned with energy optimisation objectives at the district level, while respecting operational constraints and thermal comfort requirements. This is achieved by minimising the tracking error calculated against the optimal demand profile. Additional objectives are included in the MPC, such as maximising energy efficiency or minimising costs.
Developing an MPC requires the creation of accurate predictive models of the system dynamics, which involves identifying the relevant system parameters and dynamics and formulating mathematical models that accurately capture these dynamics. These models may include physical laws and data-driven models derived from system identification techniques. The MPC must solve the optimization problem within tight time constraints to ensure real-time implementation, so the computational complexity of the models must be considered. Typically, simplified models and model order reduction techniques are used to reduce the computational cost of the problem.
To reduce the impact of model errors on performance, the MPC models are regularly updated. First, the MPC uses a technique called Moving Horizon Estimator, which updates the model with the latest measurements from the building and compensates for unpredicted phenomena (occupancy, lighting, etc.). Second, the MPC incorporates self-learning capabilities to refine and adapt the model to changes over time, including learning schemes such as recursive least squares, prediction error methods or maximum likelihood methods.
As urban areas continue to expand, the integration of Positive Energy Districts (PEDs) using Model Predictive Control (MPC) represents a significant advancement in our approach to urban energy management. MPC’s ability to predict and optimize energy consumption at the building level, in harmony with Cross-Vector Optimization strategies at the district level, enables cities to effectively harness local renewable energy sources. This integration not only enhances energy efficiency and reduces carbon emissions but also supports the resilience and sustainability of urban energy systems. Ultimately, the successful implementation of these technologies could lead to a transformative shift in how cities around the world manage and consume energy. By adopting MPC and similar technologies, cities can become more self-sufficient, reduce their environmental impact, and pave the way for a cleaner, more sustainable future.
Feel free to connect with us and share your thoughts by following us on LinkedIn and X (formerly Twitter).
Blog signed: Martin Bizkarra, Andoni Osorio (TEK)